Predictive Analytics Steers Digital Transformation Forward
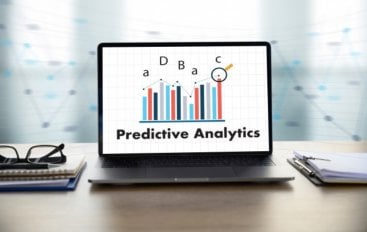
The world of business and consumer converged closer after the explosive rise of mobile technology and internet connectivity. Data became the focal point of this convergence since consumers and businesses started interacting, engaging and understanding each other through data. But managing huge volumes of unstructured data and making sense of it was a challenge for businesses. This led companies across verticals to explore various use cases in the areas of AI, Big Data, IoT and Smart technologies. With companies investing heavily on data to achieve more efficiency and performance, Gartner claims that “By 2022, 90% of corporate strategies will explicitly mention information as a critical enterprise asset and analytics as an essential competency.”
The COVID 19 pandemic has accelerated digital transformation initiatives and new investments in data analytics to close loopholes as well as strengthen businesses. But right through 2020, enterprises have been blindsided by data scarcity, and businesses are now required to operate with smaller, more varied, and multiple sources of data. There is a stronger need for forward-looking predictive analytics to deliver real-time insights. The AI/ML driven technology predictive analytics has become the central pillar of a successful digital transformation strategy.
The Promise of Predictive Analytics
Predictive Analytics, as the name suggests, predicts the future based on a deep understanding of past and present data. It uses advanced analytics and AI/ML-based algorithms to establish patterns out of historical data and then, use the very patterns to predict what is to come.
There are many use cases for Predictive Analytics across diverse verticals. For instance, asset-intensive industries such as energy, utilities and manufacturing use predictive analytics to detect machine failures in advance, while sectors like healthcare, retail and banking use AL/ML algorithms to analyse customer feedback, roll-out new products and improve customer experience.
It is worth highlighting that with the help of AI-based predictive analytics, energy industry now predicts system breakdowns and component failures with a 90% accuracy rate. Highly effective as a risk management intervention, banks apply predictive analytics to detect NPAs and circumvent frauds before they can affect their system. Organizations also use time-series models to predict future scenarios such as weather patterns, seasonal sales and traffic situations. With the capability to ascertain accurate future outcomes, predictive analytics is now welcomed by all businesses.
AI-driven Predictive Analytics For Decision-making
The natural progression for predictive analytics is to be augmented with AI capabilities. AI models make analytics more cognitive and deliver improved accuracy, predictability, and decision intelligence. The significance of AI-driven predictive analytics in the digital transformation roadmap is high because these analytical models help business leaders take effective decisions in real-time.
With large volumes of data becoming available to organizations, the data lake is paralysing business leaders and they struggle to find a single source of truth. An AI-based predictive analytics solution collects data from multiple sources, analyses patterns, culls out insights and delivers personalized suggestions through dashboards. Business leaders across functions, from finance to procurement to field operations are able to perform better and make effective decisions with the help of analytics.
Successful Adoption of Predictive Analytics
Organizations find predictive analytics highly attractive, so they rush into investments without a strategy. Unfortunately, the outcomes are not favourable as expected. As with any new strategic initiative, the change trickles from top-down. The leadership team should commit to building a data-driven business culture within the organization before making any analytics investments. The next step is to assess and confirm the consistent availability of high-quality data to feed machine learning algorithms. Without sufficient and structured data, it is next to impossible to gain any insights, and the investments will go in vain. The final critical component is to document a business plan explaining business cases (or) scenarios that need improvement with predictive analytics. The variables for each business case should be identified, measured and documented so the improvements can be measured after the implementation of the analytics solution. For instance, the leadership and analytics team should articulate if the expected business outcome is a 2% improvement in customer satisfaction or a 3% reduction in operational expenses. Successful adoption of predictive analytics also includes internal skill development to champion digital transformation.
The Future
Machine learning platforms and data science attracted significant global investments, US$229 billion, in the last 24 months. Notable investors include Sequoia Capital, Tiger Global, Goldman Sachs and Temasek. There is an evident rise in the role of predictive analytics in leading digital transformation initiatives forward. Companies of varied sizes and businesses are excited about benefiting from data intelligence.
The next couple of years will an exciting learning curve for companies that are both in the early and subsequent stages of predictive analytics adoption as they will witness the positive impact of data science.
The author is P. Rajasekaran, Chief Products Officer, Bahwan CyberTek