Brands Bring Festive Forecasting To Focal Point
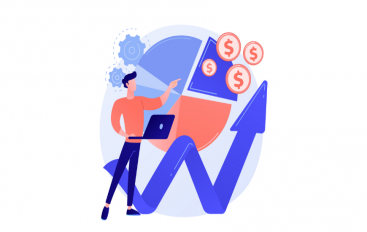
As the ongoing festival jamboree envelopes the whole of India, brands across sectors and channels are bracing for an increase in sales, decimating the ill impacts of the pandemic on businesses and behaviours. Having learnt some crucial lessons from the past year, brands are quickly actioning on the insights gathered, pushing the right promotions, ensuring stock availability for products that have been performing well, setting the right price, and ensuring the discovery of new products across multiple channels.
We all know that there is a lot that goes on behind the scenes, especially when it comes to something as large as the festive season sales. What we see on various channels is only the tip of the iceberg. When we look closely, we can see the months, if not years, of hard work that goes into making the festive sales a success. Generally, the timeline for preparing for the festive season ranges from 9 to 12 months, especially for brands who need to make a good forecast in order to begin planning.
With this, it is being observed that the traditional demand planning process is on the verge of being disrupted, as it is undergoing a significant transformation. One of the most difficult challenges this process faces is gaining insights from the massive amount of untapped data that exists in various pockets. “Due to a large number of redundant tasks and the fact that multiple parties must align, the time it takes today for demand planners to generate forecasts and plans is inefficient. In India, the sheer number of SKUs, various channels, and market volatility makes forecasting and planning in a short period of time extremely difficult. By making data-driven decisions, this process can be optimised to make the supply chain more responsive. With the rise of AI and ML technologies, demand planners now have access to incredibly powerful tools and insights,” asserts Rahul Vishwakarma, Co-founder and CEO, Mate Labs.
AI-Powered Forecasting Tools Gaining Prominence
Demand forecasting has become the most commonly used machine learning application in supply chain planning, according to a recent Gartner’s survey. It says that 45% of businesses are already utilising the technology, and 43% intend to use AI-powered demand forecasting within the next two years. Machine learning algorithms increase the accuracy of forecasting methods and optimise replenishment processes. Companies are reducing the cost of cash-in-hand and out-of-stock scenarios as a result of these advancements.
Unlike traditional tools, integrating AI and ML is enabling brands to comprehend a wide range of real-time inventory control dynamics that influence inventory stock levels. It is also assisting brands in predicting various scenarios and recommending actions - either autonomously or with human approval.
“The technology consolidates, standardises, and enriches data, laying the groundwork for AI analytics to present data-driven recommendations to managers. Its ability to act autonomously can free up personnel time spent on routine tasks, allowing managers and business owners to focus on more strategic decision-making,” adds Vishwakarma.
Drawing a parallel between the pre and post-pandemic era, Rajat Sharma, Vice President, ISCM & Customer Service, Hamilton Housewares observes customers having similar purchasing patterns before the crisis hit, which made the demand forecasting models much easier to use. However, with the onset of the pandemic, he sees a significant shift in consumer behaviour, compelling marketers to track and record such dynamics through an advanced technology that is swift and accurate.
“During the Covid-period, we observed two types of consumers. One, certain customers' purchasing power had decreased, along with their per unit purchasing or per product spend, affecting certain product categories. These customers began looking for less expensive options and were willing to sacrifice features, form, and even a certain level of quality. Second, there were customers with spending power who were motivated to buy better and safer quality products due to their fear of COVID,” he adds.
Hamilton was quick to comprehend how consumers progressed to a higher level of lifestyle, how they began to adopt healthier and safer practices. As a result, the company significant increase in demand for thermoflasks for consumers to store/carry/consume hot water. It would have missed out on a huge opportunity if we had not been ready to tap into this consumer behaviour. Hence, it is critical for supply chain management to understand consumer behaviour across channels, product categories, geographies, etc., in order to create a sophisticated information grid. This helps in determining which segments to invest in and what volume will sell in the market.
Giving us a sense of how these forecasting tools are augmenting growth in retail fashion, Ganesan V.P., Distribution Sector Leader, IBM Consulting, India/South Asia takes the case of fast fashion specifically, which has disrupted the traditional fashion seasons that were being followed by the entire industry. “Since successful fast fashion business models deliver new products every two weeks i.e., 26 times a year, rather than typical, fall, winter, spring and summer seasons and have a typical design-to-retail cycle of about five weeks, the rapid rate at which new capsule collections and trendy designs are being released only feeds into shoppers’ desire to buy more.
There is a lot of important data that is available within an enterprise already in the form of sales data, design data and customer experience data within the ERP, CRM, PLM, SCM tools which we spoke about earlier. AI can help analyse the patterns hidden within this data and make sense out of it. It can tell the brand which design sold well, where and how fast it sold. AI can now go a step further and predict why a particular product or design sold well or did not sell. With Computer Vision, they can marry transactional data with visual referencing to analyse the look of apparel and predict its sell-through rate,” he says.
Bestseller India, the fashion company which owns brands such as Jack & Jones, Vero Moda & others, uses IBM Garage methods to develop Fabric.ai, India’s first AI-powered platform for the fashion industry. Fabric.ai derives insights from data to forecast demand more accurately, sell more apparel at higher margins and reduce unsold inventory. By infusing intelligence across its products and processes, the company built a smarter, more resilient business.
Bestseller also leverages IBM Watson’s AI capability on the cloud to predict the right merchandise for the consumer at the right time. With Watson mining deeply into big data, the retailer can determine the right assortment plan for each store, predict the next best product to incorporate into its mix, and improve the efficiency of its supply chain.
The Festive-Focussed Approach
We realise how demand forecasting is crucial for all business types, perhaps more so than ever during this festive time, which has arrived after a prolonged break. Every facet of the company operations is affected by these forecasts, helping make better business decisions. Brands are reaping the many benefits of the AI/ML technologies and tools through the below ways:
● To design new products - With intelligence on how people use a product, AI can assist brands in understanding how to design, test, produce, market, and launch specific products that perform better in the market. This technology can save a company millions in R&D costs if it is heavily reliant on manufacturing. It is so because the company will waste less time and resources, which is always a plus
● To reduce the planning cycle - Brands that are launching new products during the festive season typically have long planning cycles and struggle to change plans in response to real-time events. The planning cycle for the manufacture, marketing, shipping, and sale of a new product could take weeks or months using traditional planning techniques. Integrating AI and ML allows planners to prepare for unexpected changes, particularly during the festive season, and to further optimise their inventory and supply chain, resulting in higher revenue growth. The goal here is to shorten planning cycles and the time it takes to change those plans so that they are as close to the point of action as possible
● To improve stock availability and avoid overstocking - It is possible to improve demand sensing and forecast accuracy by combining all available data sources, including customer sentiment analysis, with supervised machine learning algorithms. ML algorithms can correlate location-specific sentiment for a given product or brand with the regional availability of that product. Having this knowledge alone can save the retail industry up to $50 billion in obsolete inventory each year
● To push customised shopping recommendations - To create a real-time personalisation experience, brands today rely on predictive analytics and machine learning. E-commerce companies, in particular, can use the collected and processed data to recommend personalised products to each customer in real-time, creating an individual, user-oriented shopping experience. AI solutions in online retail create a customer journey that is uniquely tailored to each consumer's interests and needs, going far beyond simply purchasing a product
● To enable dynamic pricing - Because of its ability to adjust and reoptimise based on variables such as inventory levels, number of add to carts, and product-based sentiment analysis, machine learning has emerged as the modern solution to dynamic pricing. Predictive analytics allows brands to input variables they want to see, such as a final revenue result or inventory levels at zero, and forecast how to get there given the current price optimisation model
● To set the right promotional strategy and offers - Brands that use AI and ML are better equipped to provide personalised offers to their customers, and discounts, coupons, and recommended products are presented through modern digital marketing tactics such as email promotions and in-app offers. Customers are more likely to act on offers tailored specifically to them, increasing a brand's ROI
● To create easy product discovery - Showing the right products to the right users at the right time is the key to successful e-commerce product discovery. With AI and ML solutions, you can make this process intuitive and simple for your online visitors, regardless of how they shop. You can make customers feel heard and understood by providing personalised product discovery. This strengthens brand affinity and enhances the customer experience
As time and businesses evolve, AI and ML will be able to tell you where the ROI will come from with this type of sale, which categories are performing well, where the capacities are being utilised, and where the next investments should be made. Integrating AI and machine learning into your supply chain solutions will also make repetitive and mundane tasks easier. It will soon begin to interact with IoT devices, whether they are sensors, boosters, or production technology on the floor, sum experts.